GC Data Conference 2023/Discover more about data
vExpo | Agenda | Speakers | Networking | Discover more about data 2022 |
The 2023 GC Data Conference is brought to you by Innovation, Science and Economic Development Canada and the Canada School of Public Service with support from the GC Data Community.
Discover more about data 2023
Are we missing something? Send us the details: GC Data Community
Learning
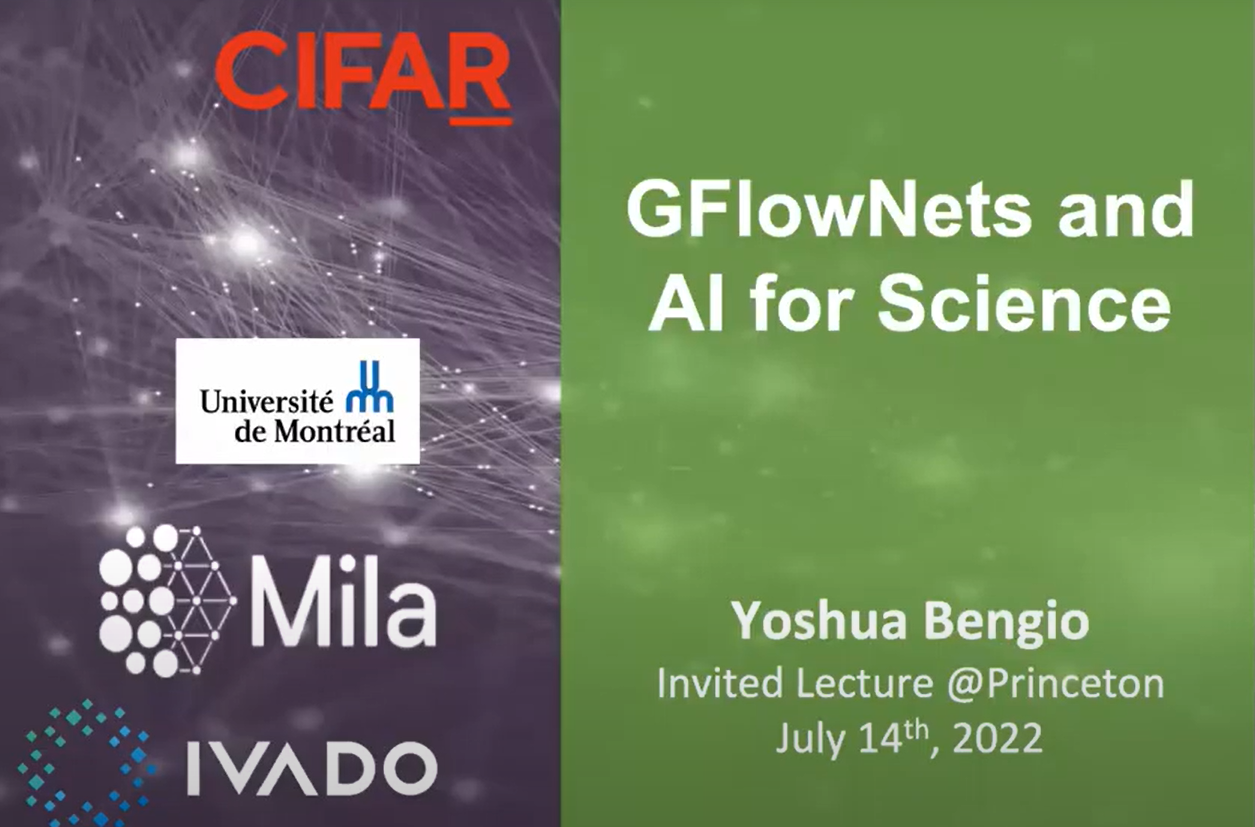
GFlowNets and AI for Science presentation - Princeton AI Club
(In English) Machine learning research is expanding its reach, beyond the traditional realm of the tech industry and into the activities of other scientists, opening the door to truly transformative advances in these disciplines. In this talk I will focus on two aspects, modeling and experimental design, that are intertwined in the theory-experiment-analysis active learning loop that constitutes a core element of the scientific methodology. Computers will be necessary to go beyond the currently purely manual research loop and take advantage of high-throughput experimental setups and large-scale experimental datasets. I will introduce a novel machine learning framework called GFlowNets (for “Generative Flow Networks”), related to reinforcement learning, generative modeling and variational methods and conceived as an ML-driven replacement for MCMC. GFlowNets were first used to propose a highly diverse set of molecular candidates and were then incorporated in an active learning framework for efficiently looking for molecules with desirable properties. More recently, we have been exploring how GFlowNets can generate not just molecular graphs but also causal graphs and Bayesian posterior distributions in function space. I will describe our research program to build on these bases and develop machine learning methodologies for efficiently exploring the space of causal theories as well as the space of experiments while characterizing the ambiguities left by finite datasets and non-identifiability, as well as our plans to apply these tools in areas of great societal need like the unmet challenge of antimicrobial resistance.
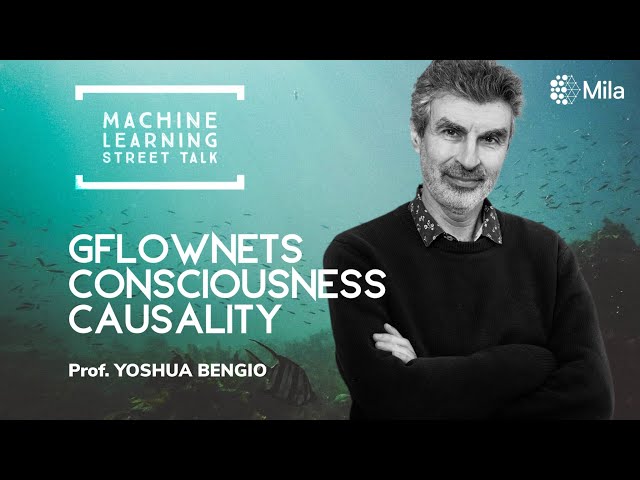
GFlowNets, Consciousness & Causality - Machine Learning Street Talk
(In English) For Yoshua Bengio, GFlowNets are the most exciting thing on the horizon of Machine Learning today. He believes they can solve previously intractable problems and hold the key to unlocking machine abstract reasoning itself. This discussion explores the promise of GFlowNets and the personal journey Prof. Bengio traveled to reach them.
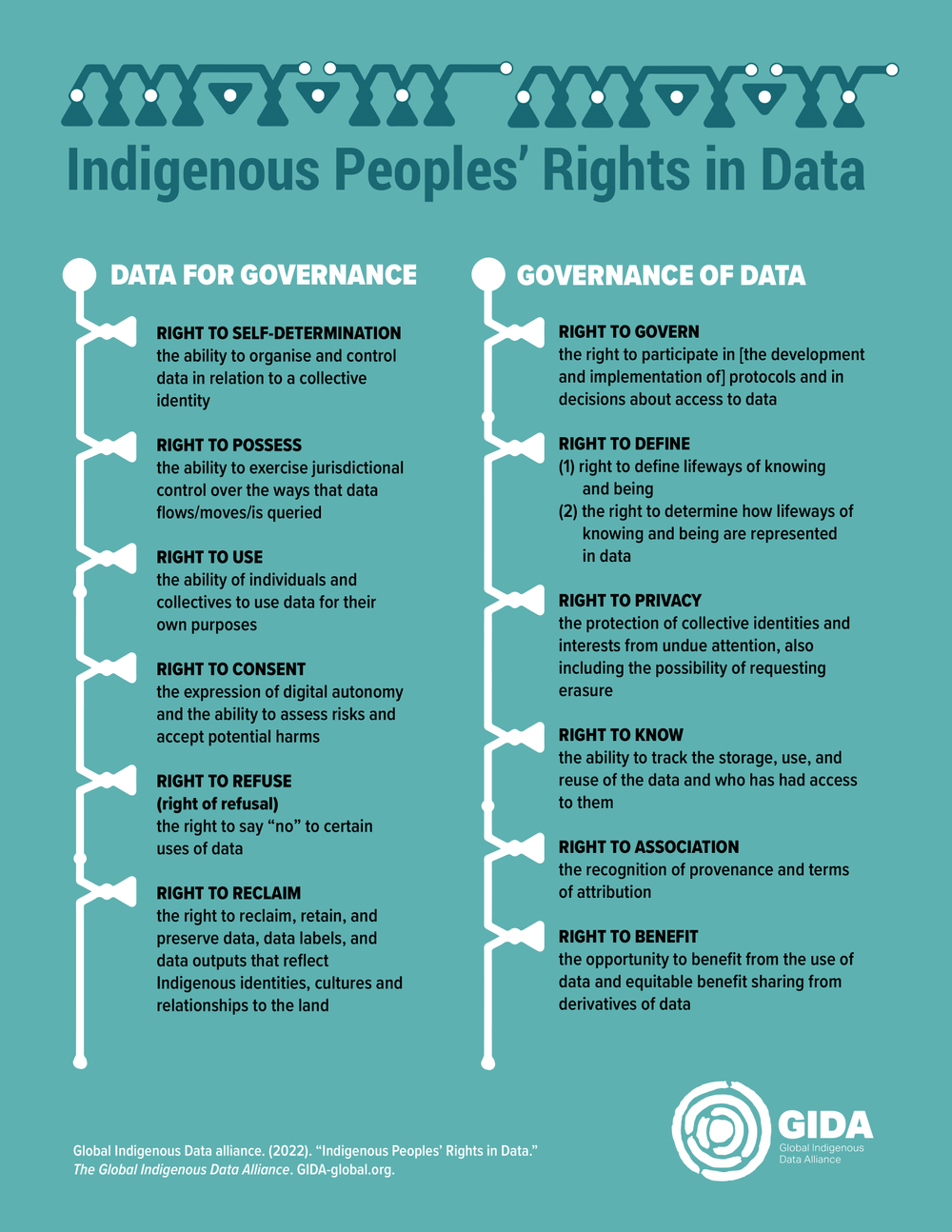
Indigenous Peoples’ Rights in Data
(In English) Global Indigenous Data Alliance (GIDA) has developed a set of rights for Indigenous peoples’ rights in data.
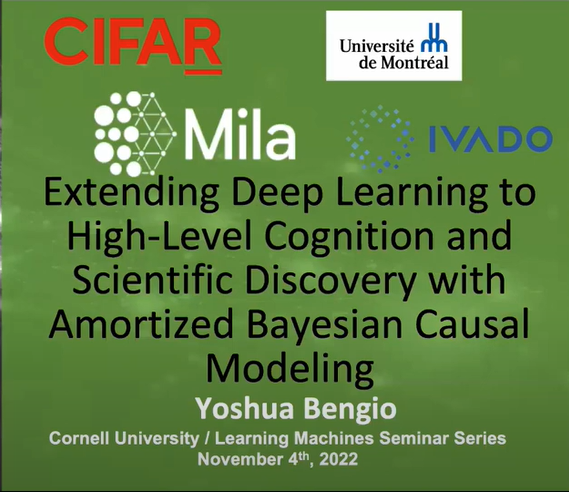
Learning Machines Seminar: Extending Deep Learning to High-Level Cognition and Scientific Discovery with Amortized Bayesian Causal Modeling
(In English) How can what has been learned on previous tasks generalize quickly to new tasks or changes in distribution? The study of conscious processing in human brains (and the window into it given by natural language) suggests that we are able to decompose high-level verbalizable knowledge into reusable components (roughly corresponding to words and phrases). This has stimulated research in modular neural networks where attention mechanisms can be used to dynamically select which modules should be brought to bear in a given new context. Another source of inspiration for tackling this challenge is the body of research into causality, where changes in tasks and distributions are viewed as interventions. The crucial insight is that we need to learn to separate (somewhat like in meta-learning) what is stable across changes in distribution, environments or tasks and what may be separate to each of them or changing in non-stationary ways in time. From a causal perspective what is stable are the reusable causal mechanisms, along with the inference machinery to make probabilistic guesses about the appropriate combination of mechanisms (maybe seen as a graph) in a particular new context. What may change with time are the interventions and other random variables which are those that yield more directly to observations. If interventions are not observed (we do not have labels for fully explaining the changes in tasks in terms of the underlying modules and causal variables) we would ideally like to estimate the Bayesian posterior over the interventions, given whatever is observed. This research approach raises many interesting research questions ranging from Bayesian inference and identifiability to causal discovery, representation learning and out-of-distribution generalization and adaptation, which will be discussed in the presentation.
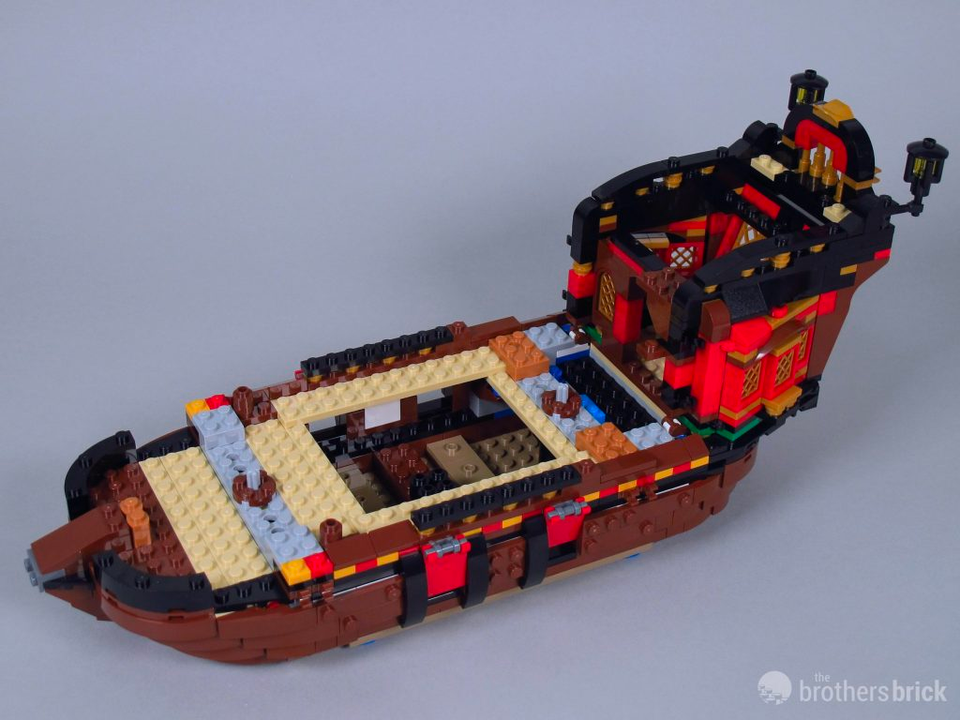
The GFlowNet Tutorial
(In English) A GFlowNet is a trained stochastic policy or generative model, trained such that it samples objects x through a sequence of constructive steps, with probability proportional to a reward function R(x), where R is a non-negative integrable function. This makes a GFlowNet able to sample a diversity of solutions x that have a high value of R(x).
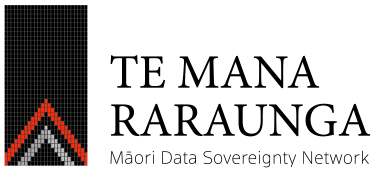
Principles of Māori Data Sovereignty
(In English) This Te Mana Raraunga (TMR) Brief provides a general overview of key Māori Data Sovereignty terms and principles.
Events and opportunities to get involved
Consultation Standard on Managing Metadata (GCpedia)
A new Standard on Managing Metadata is being developed to replace and expand on the Standard on Metadata. This new standard is meant to look beyond the use of metadata to support information management and capture requirements that will ensure the strategic, efficient, and effective management of metadata for both information and data across the GC. You can share your ideas, comments and feedback to ensure that the Standard on Managing Metadata is complete, correct, understandable, useful, and realistic. Send us your feedback before March 10.
Data for Impact Series: Building Powerful Data Hubs (link coming soon)
As the first event of the Data for Impact Series, this event will showcase how data hubs are being used within the public service and how they support the renewed federal Data Strategy.
March 29, 2023 | 1 hour | Webcast
Books and reports
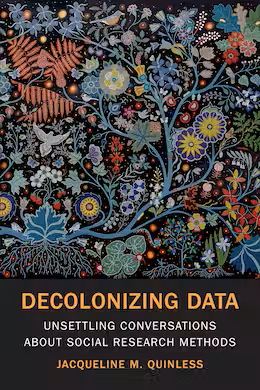
Decolonizing Data: Unsettling Conversations about Social Research Methods
(In English) Decolonizing Data explores how ongoing structures of colonialization negatively impact the well-being of Indigenous peoples and communities across Canada, resulting in persistent health inequalities. In addressing the social dimensions of health, particularly as they affect Indigenous peoples and BIPOC communities, Decolonizing Data asks, Should these groups be given priority for future health policy considerations? Decolonizing Data provides a deeper understanding of the social dimensions of health as applied to Indigenous peoples, who have been historically underfunded in and excluded from health services, programs, and quality of care; this inequality has most recently been seen during the COVID-19 pandemic. Drawing on both western and Indigenous methodologies, this unique scholarly contribution takes both a sociological perspective and the "two-eyed seeing" approach to research methods. By looking at the ways that everyday research practices contribute to the colonization of health outcomes for Indigenous peoples, Decolonizing Data exposes the social dimensions of healthcare and offers a careful and respectful reflection on how to "unsettle conversations" about applied social research initiatives for our most vulnerable groups.
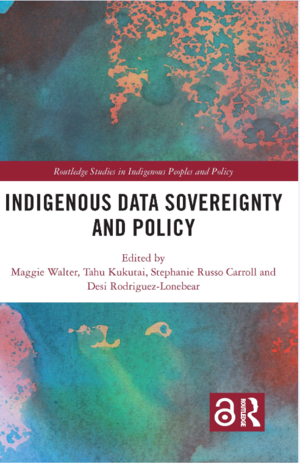
Indigenous Data Sovereignty and Policy
(In English) This book examines how Indigenous Peoples around the world are demanding greater data sovereignty, and challenging the ways in which governments have historically used Indigenous data to develop policies and programs.
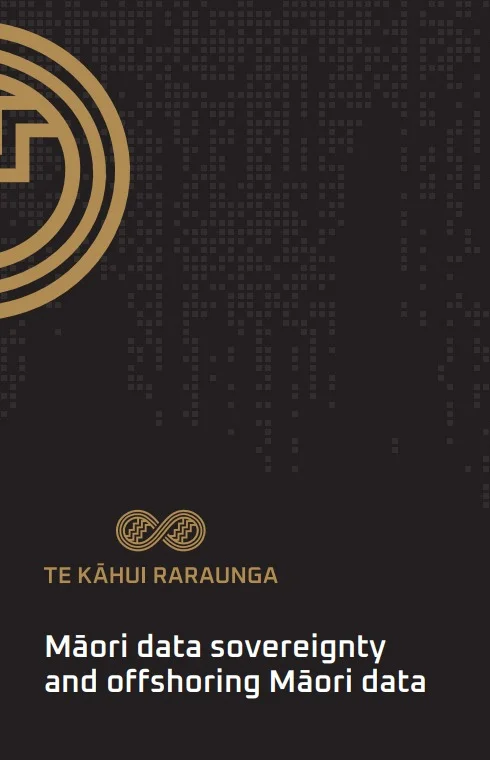
Māori data sovereignty and offshoring Māori data
(In English) Government agencies in Aotearoa New Zealand are increasingly offshoring their data, citing greater security and reduced cost as key factors.
As the government accelerates its digital transformation strategy across the public service, Māori data sovereignty requirements must be central to decision making, particularly with regard to offshoring and procurement.
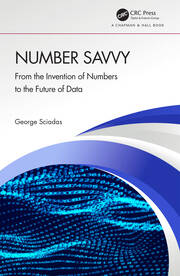
Number Savvy - From the Invention of Numbers to the Future of Data
(In English) This book is written for the love of numbers. It tells their story, shows how they were invented and used to quantify our world, and explains what quantitative data mean for our lives. It aspires to contribute to overall numeracy through a tour de force presentation of the production, use, and evolution of data.Understanding our physical world, our economies, and our societies through quantification has been a persistent feature of human evolution. This book starts with a narrative on why and how our ancestors were driven to the invention of number, which is then traced to the eventual arrival at our number system. This is followed by a discussion of how numbers were used for counting, how they enabled the measurement of physical quantities, and how they led to the estimation of man-made and abstract notions in the socio-economic domain. As data don’t fall like manna from the sky, a unique feature of this book is that it explains from a teacher’s perspective how they’re really conceived in our minds, how they’re actually produced from individual observations, and how this defines their meaning and interpretation. It discusses the significance of standards, the use of taxonomies, and clarifies a series of misconceptions regarding the making of data. The book then describes the switch to a new research paradigm and its implications, highlights the arrival of microdata, illustrates analytical uses of data, and closes with a look at the future of data and our own role in it.
Are we missing something? Send us the details: GC Data Community
GC Data Community | Contact us | Subscribe | Join us on GCcollab | GC Data Community Partners