Important: The GCConnex decommission will not affect GCCollab or GCWiki. Thank you and happy collaborating!
Difference between revisions of "Using AI to advance oceanography"
(4 intermediate revisions by the same user not shown) | |||
Line 3: | Line 3: | ||
− | AI can provide a data-driven approach to analyzing ocean data. Department of Fisheries and Oceans (DFO) has developed a predictive model to sift through the | + | AI can provide a data-driven approach to analyzing ocean data. Department of Fisheries and Oceans (DFO) has developed a predictive model to sift through the piles of ocean data to find (dis-)similarities between multidimensional profiles of oceanographic data. '''The insights gained from the model can be used to answer any questions about dynamic changes in our oceans.''' |
− | |||
− | |||
Line 14: | Line 12: | ||
+ | |||
Line 27: | Line 26: | ||
+ | |||
+ | |||
== The Solution == | == The Solution == | ||
− | |||
[[File:Ocean Profile Classification Model.png|alt=Data-driven approach for analyzing ocean data|thumb|483x483px|<small>'''Profile classification model: a data-driven approach for analyzing ocean data'''</small> <ref>Guillaume Maze et al, Coherent heat patterns revealed by unsupervised classification of Argo temperature profiles in the North Atlantic Ocean, Progress in Oceanography, Volume 151, 2017, Pages 275-292.</ref>]] | [[File:Ocean Profile Classification Model.png|alt=Data-driven approach for analyzing ocean data|thumb|483x483px|<small>'''Profile classification model: a data-driven approach for analyzing ocean data'''</small> <ref>Guillaume Maze et al, Coherent heat patterns revealed by unsupervised classification of Argo temperature profiles in the North Atlantic Ocean, Progress in Oceanography, Volume 151, 2017, Pages 275-292.</ref>]] | ||
+ | Data-driven analysis approaches are better suited for such type of analysis. AI can sift through the piles of ocean data to find the complex relationship between the ocean observations. | ||
+ | Supported by the 2020 – 2021 Results Fund, a proof-of-concept was developed for a predictive model to find (dis-)similarities between in situ multidimensional profiles of oceanographic data of the Pacific Ocean. The in situ Conductivity, Temperature, Depth (CTD) profiles are classified using the profile clustering model <ref name=":0">https://pyxpcm.readthedocs.io/en/latest/index.html</ref>. The model automatically assembles ocean profiles into clusters according to their vertical structure similarities. The geospatial properties of these clusters can be used to address a large variety of oceanographic problems, e.g., front detection, water mass identification, natural region contouring (gyres, eddies), reference profile selection for quality control validation, etc. The vertical structure of these clusters furthermore provides a highly synthetic representation of large ocean areas that can be used for dimensionality reduction and coherent intercomparisons of ocean data (re)-analysis or simulations <ref name=":0" />. | ||
− | + | We have applied the predictive model to cluster a total of 3602 CTD profiles spanning from 2000 to 2019, using their temperature and salinity values. Initial results have shown that the dataset contains 9 groups of vertically coherent classes. Each of the classes reveals unique and physically coherent heat distributions along the vertical axis. When mapped in space, each of the 9 classes is found to define an oceanic region, even though no spatial information was used in the model determination. The model is also able to show natural phenomena such as ocean inlets, shown as purple observations in the figure below. | |
+ | [[File:Ocean data before after.png|alt=The ocean profiles before and after applying the predictive model|center|thumb|1114x1114px|'''<small>The ocean profiles before and after applying the predictive model</small>''']] | ||
+ | In addition, there is a possibility of identifying anomalous profiles by examining how much an ocean profile deviates from its cluster. In the figure below, results have shown that there is a total of 4 profiles that may be anomalous. Those identified profiles belong to clusters 0, 4, and 6. | ||
+ | [[File:Anomlous profiles.png|center|thumb|531x531px|<small>'''Applying the predictive model to identify anomalous profiles.'''</small>]] | ||
+ | In the end, the model can provide ocean scientists with a data-driven tool to analyze ocean data. | ||
− | + | == Next Steps == | |
− | + | With the functionality of potential benefits of the model now established from the proof-of-concept stage, the next step is to deploy the model in a field testing environment to explore the business value it can provide in a real-world setting. Similar to the CTD quality control model, this model is planned to be deployed via a real-time endpoint on a cloud analytics platform. Model communications will be managed through a client-side program and the model results will be delivered via an interactive Power BI dashboard. This architecture will enable users to easily send new data to the model for processing and explore the results in interactive maps with data annotations. | |
− | |||
− | |||
− | |||
== References == | == References == |
Latest revision as of 17:48, 22 December 2022
(Français: L’IA au service de l’océanographie)
AI can provide a data-driven approach to analyzing ocean data. Department of Fisheries and Oceans (DFO) has developed a predictive model to sift through the piles of ocean data to find (dis-)similarities between multidimensional profiles of oceanographic data. The insights gained from the model can be used to answer any questions about dynamic changes in our oceans.
The Challenge

DFO Canada has been surveying Canada’s oceans to monitor the evolution of Canada’s oceans, as well as to perform scientific research. The department frequently collects ocean observations using in situ measurements. Ocean data is considered multidimensional data where ocean observations are collected at different depths of the ocean. The amount of ocean data and data dimensions is rising sharply. Scientists tried to use simulations to simulate the ocean environment. However, ocean simulation models don’t reflect the complex relationship between the different ocean observations. Moreover, current traditional ocean data analysis mostly uses manual classification and recognition. This can be resource-intensive, time-consuming, and requires a specific kind of expertise.
The Solution
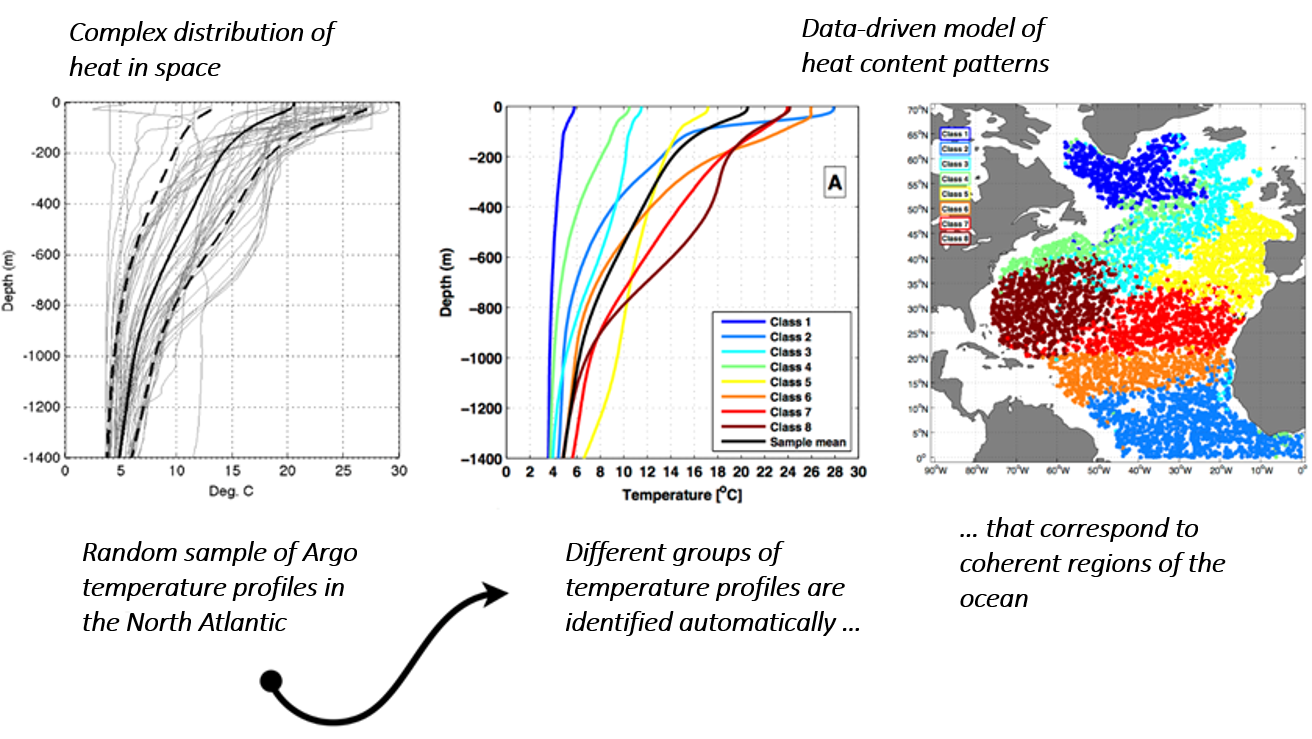
Data-driven analysis approaches are better suited for such type of analysis. AI can sift through the piles of ocean data to find the complex relationship between the ocean observations.
Supported by the 2020 – 2021 Results Fund, a proof-of-concept was developed for a predictive model to find (dis-)similarities between in situ multidimensional profiles of oceanographic data of the Pacific Ocean. The in situ Conductivity, Temperature, Depth (CTD) profiles are classified using the profile clustering model [3]. The model automatically assembles ocean profiles into clusters according to their vertical structure similarities. The geospatial properties of these clusters can be used to address a large variety of oceanographic problems, e.g., front detection, water mass identification, natural region contouring (gyres, eddies), reference profile selection for quality control validation, etc. The vertical structure of these clusters furthermore provides a highly synthetic representation of large ocean areas that can be used for dimensionality reduction and coherent intercomparisons of ocean data (re)-analysis or simulations [3].
We have applied the predictive model to cluster a total of 3602 CTD profiles spanning from 2000 to 2019, using their temperature and salinity values. Initial results have shown that the dataset contains 9 groups of vertically coherent classes. Each of the classes reveals unique and physically coherent heat distributions along the vertical axis. When mapped in space, each of the 9 classes is found to define an oceanic region, even though no spatial information was used in the model determination. The model is also able to show natural phenomena such as ocean inlets, shown as purple observations in the figure below.
In addition, there is a possibility of identifying anomalous profiles by examining how much an ocean profile deviates from its cluster. In the figure below, results have shown that there is a total of 4 profiles that may be anomalous. Those identified profiles belong to clusters 0, 4, and 6.
In the end, the model can provide ocean scientists with a data-driven tool to analyze ocean data.
Next Steps
With the functionality of potential benefits of the model now established from the proof-of-concept stage, the next step is to deploy the model in a field testing environment to explore the business value it can provide in a real-world setting. Similar to the CTD quality control model, this model is planned to be deployed via a real-time endpoint on a cloud analytics platform. Model communications will be managed through a client-side program and the model results will be delivered via an interactive Power BI dashboard. This architecture will enable users to easily send new data to the model for processing and explore the results in interactive maps with data annotations.
References
- ↑ https://argo.ucsd.edu/about/
- ↑ Guillaume Maze et al, Coherent heat patterns revealed by unsupervised classification of Argo temperature profiles in the North Atlantic Ocean, Progress in Oceanography, Volume 151, 2017, Pages 275-292.
- ↑ Jump up to: 3.0 3.1 https://pyxpcm.readthedocs.io/en/latest/index.html